Commercial Hostile Influence Networks: Anthropic’s Findings on Multi-Client Operations
- CRC
- May 9
- 3 min read
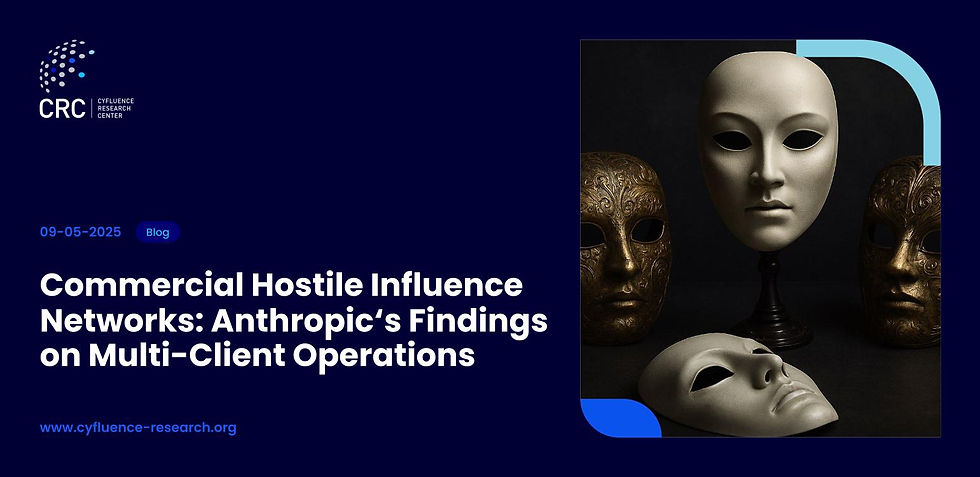
[Introduction]
In April 2025, the company Anthropic published an analysis of four coordinated influence operations, which it claims to have disrupted. They were carried out by a commercial provider ("Influence-as-a-Service") and could not be attributed to any specific state actor. (1)
[Technical Implementation of the Campaigns]
Claude, a large language model (LLM) developed by Anthropic, was used for content generation and managing digital identities. Based on predefined parameters, the model determined how controlled online personas should respond to posts—whether to comment, share, like, or ignore them.
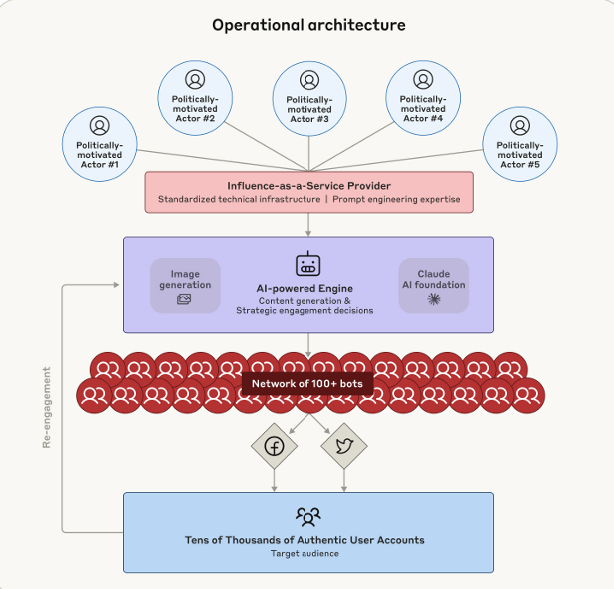
The coordination was managed through a JSON-based framework that defined each persona’s political alignment, language style, and behavioral patterns. Claude also created multilingual content, generated prompts for image-generation models, and evaluated image outputs for narra-tive alignment. This enabled the automated, cross-platform, and consistent management of over 100 social media accounts. (3)
[Campaign Targets and Regional Focus]
The four documented operations were directed at different regions. In Europe, the focus was on energy-related narratives; in Iran, on cultural identity. In the case of the United Arab Emirates, the content emphasized economic strength while criticizing EU regulations. In Kenya, political develop-ment initiatives were positively portrayed. The operation targeted opposition figures in one EU member state while promoting Albanian political personalities. Despite differing objectives, the technical execution was uniform across all campaigns. (4)
[Communication Strategy and Impact]
According to Anthropic, only a limited viral impact was reached. The goal was not rapid viral spread, but the long-term embedding of credible-appearing personas within ongoing digital conversations. The accounts were designed to appear authentic. To simulate human behavior, the personas responded to bot accusations from real users with pre-scripted sarcasm or deflection, reinforcing the impression of genuine interaction. (5)
[Conclusion]
LLM models will remain structurally vulnerable. Conventional filters are insufficient to detect long-term, coordinated manipulation. Preventing misuse remains one of the central challenges in managing the risks associated with scalable AI systems.
The report underscores the growing role of private actors in political manipulation. Unlike state entities, these providers operate without ideological alignment or institutional oversight. They act opportunistically, adapt to client needs, and deploy general-purpose AI systems with standardized infrastructure to carry out large-scale influence operations, even with limited resources.
The rising demand has given rise to an "Influence-as-a-Service" sector that offers modular, low-cost tools for outsourced political messaging while minimizing clients' attribution risks. This development has turned influence into a technically optimized, professionalized, scalable service detached from ideology.
Anthropic’s findings show that individual operators can simultaneously serve conflicting geopolitical interests, accelerating an evolution in such campaigns' reach, precision, and impact. It seems that tactics are shifting: rather than chasing virality, actors increasingly prioritize the stealthy construction of long-lasting, embedded networks that gradually shape discourse through persistent and believable engagement.
This strategic pivot makes influence operations harder to detect and disrupt. Addressing this challenge will require strong national policy frameworks and active cooperation from developers and providers of large language models, who play a key role in identifying misuse and enforcing safeguards at scale.
Footnotes
(1) Anthropic, K. Lebedev, A. Moix & J. Klein, 2025. Operating Multi-Client Influence Networks Across Platforms. p.1 [PDF]
Available at:
(2) Anthropic, K. Lebedev, A. Moix & J. Klein, 2025. Operating Multi-Client Influence Networks Across Platforms. p.3 [PDF]
Available at:
(3) Anthropic, K. Lebedev, A. Moix & J. Klein, 2025. Operating Multi-Client Influence Networks Across Platforms. p.2 [PDF]
Available at:
(4) Anthropic, K. Lebedev, A. Moix & J. Klein, 2025. Operating Multi-Client Influence Networks Across Platforms. p.2 [PDF]
Available at:
(5) Anthropic, K. Lebedev, A. Moix & J. Klein, 2025. Operating Multi-Client Influence Networks Across Platforms. p.2 [PDF]
Available at: